What is Descriptive Statistics? Definition, Types, Examples
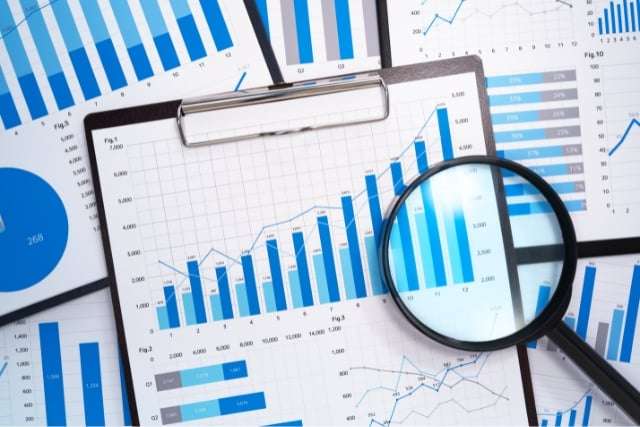
Have you ever wondered how we make sense of the vast sea of data surrounding us? In a world overflowing with information, the ability to distill complex datasets into meaningful insights is a skill of immense importance. This guide will equip you with the knowledge and tools to unravel the stories hidden within data. Whether you're a data analyst, a researcher, a business professional, or simply curious about the art of data interpretation, this guide will demystify the fundamental concepts and techniques of descriptive statistics, empowering you to explore, understand, and communicate data like a seasoned expert.
What is Descriptive Statistics?
Descriptive statistics refers to a set of mathematical and graphical tools used to summarize and describe essential features of a dataset. These statistics provide a clear and concise representation of data, enabling researchers, analysts, and decision-makers to gain valuable insights, identify patterns, and understand the characteristics of the information at hand.
Purpose of Descriptive Statistics
- Data Summarization: They provide a compact summary of the main characteristics of a dataset, allowing individuals to grasp the essential features quickly.
- Data Visualization: Descriptive statistics often accompany visual representations, such as histograms, box plots, and bar charts, making it easier to interpret and communicate data trends and distributions.
- Data Exploration: They facilitate the exploration of data to identify outliers, patterns, and potential areas of interest or concern.
- Data Comparison: Descriptive statistics enable the comparison of datasets, groups, or variables, aiding in decision-making and hypothesis testing.
- Informed Decision-Making: By providing a clear understanding of data, descriptive statistics support informed decision-making across various domains, including business, healthcare, social sciences, and more.
Importance of Descriptive Statistics in Data Analysis
Descriptive statistics play a pivotal role in data analysis by providing a foundation for understanding, summarizing, and interpreting data. Their importance is underscored by their widespread use in diverse fields and industries.
Here are key reasons why descriptive statistics are crucial in data analysis:
- Data Simplification: Descriptive statistics simplify complex datasets, making them more accessible to analysts and decision-makers. They condense extensive information into concise metrics and visual representations.
- Initial Data Assessment: Descriptive statistics are often the first step in data analysis. They help analysts gain a preliminary understanding of the data's characteristics and identify potential areas for further investigation.
- Data Visualization: Descriptive statistics are often paired with visualizations, enhancing data interpretation. Visual representations, such as histograms and scatter plots, provide intuitive insights into data patterns.
- Communication and Reporting: Descriptive statistics serve as a common language for conveying data insights to a broader audience. They are instrumental in research reports, presentations, and data-driven decision-making.
- Quality Control: In manufacturing and quality control processes, descriptive statistics help monitor and maintain product quality by identifying deviations from desired standards.
- Risk Assessment: In finance and insurance, descriptive statistics, such as standard deviation and variance, are used to assess and manage risk associated with investments and policies.
- Healthcare Decision-Making: Descriptive statistics inform healthcare professionals about patient demographics, treatment outcomes, and disease prevalence, aiding in clinical decision-making and healthcare policy formulation.
- Market Analysis: In marketing and consumer research, descriptive statistics reveal customer preferences, market trends, and product performance, guiding marketing strategies and product development.
- Scientific Research: In scientific research, descriptive statistics are fundamental for summarizing experimental results, comparing groups, and identifying meaningful patterns in data.
- Government and Policy: Government agencies use descriptive statistics to collect and analyze data on demographics, economics, and social trends to inform policy decisions and resource allocation.
Descriptive statistics serve as a critical foundation for effective data analysis and decision-making across a wide range of disciplines. They empower individuals and organizations to extract meaningful insights from data, enabling more informed and evidence-based choices.
Data Collection and Preparation
First, let's delve deeper into the crucial initial data collection and preparation steps. These initial stages lay the foundation for effective descriptive statistics.
Data Sources
When embarking on a data analysis journey, you must first identify your data sources. These sources can be categorized into two main types:
- Primary Data: This data is collected directly from original sources. It includes surveys, experiments, and observations tailored to your specific research objectives. Primary data offers high relevance and control over the data collection process.
- Secondary Data: Secondary data, on the other hand, is data that already exists and has been collected by someone else for a different purpose. It can include publicly available datasets, reports, and databases. Secondary data can save time and resources but may not always align perfectly with your research needs.
Data Types
Understanding the nature of your data is fundamental. Data can be classified into two primary types:
- Quantitative Data: Quantitative data consists of numeric values and is often used for measurements and calculations. Examples include age, income, temperature, and test scores. Quantitative data can further be categorized as discrete (countable) or continuous (measurable).
- Qualitative Data: Qualitative data, also known as categorical data, represents categories or labels and cannot be measured numerically. Examples include gender, color, and product categories. Qualitative data can be nominal (categories with no specific order) or ordinal (categories with a meaningful order).
Data Cleaning and Preprocessing
Once you have your data in hand, preparing it for analysis is essential. Data cleaning and preprocessing involve several critical steps:
Handling Missing Data
Missing data can significantly impact your analysis. There are various approaches to address missing values:
- Deletion: You can remove rows or columns with missing data, but this may lead to a loss of valuable information.
- Imputation: Imputing missing values involves estimating or filling in the missing data using methods such as mean imputation, median imputation, or advanced techniques like regression imputation.
Outlier Detection
Outliers are data points that deviate significantly from the rest of the data. Detecting and handling outliers is crucial to prevent them from skewing your results. Popular methods for identifying outliers include box plots and z-scores.
Data Transformation
Data transformation aims to normalize or standardize the data to make it more suitable for analysis. Common transformations include:
- Normalization: Scaling data to a standard range, often between 0 and 1.
- Standardization: Transforming data to have a mean of 0 and a standard deviation of 1.
Data Organization and Presentation
Organizing and presenting your data effectively is essential for meaningful analysis and communication. Here's how you can achieve this:
Data Tables
Data tables are a straightforward way to present your data, especially when dealing with smaller datasets. They allow you to list data in rows and columns, making it easy to review and perform basic calculations.
Graphs and Charts
Visualizations play a pivotal role in conveying the message hidden within your data. Some common types of graphs and charts include:
- Histograms: Histograms display the distribution of continuous data by dividing it into intervals or bins and showing the frequency of data points within each bin.
- Bar Charts: Bar charts are excellent for representing categorical or discrete data. They display categories on one axis and corresponding values on the other.
- Line Charts: Line charts are useful for identifying trends over time, making them suitable for time series data.
- Scatter Plots: Scatter plots help visualize the relationship between two variables, making them valuable for identifying correlations.
- Pie Charts: Pie charts are suitable for displaying the composition of a whole in terms of its parts, often as percentages.
Summary Statistics
Calculating summary statistics, such as the mean, median, and standard deviation, provides a quick snapshot of your data's central tendencies and variability.
When it comes to data collection and visualization, Appinio offers a seamless solution that simplifies the process. In Appinio, creating interactive visualizations is the easiest way to understand and present your data effectively. These visuals help you uncover insights and patterns within your data, making it a valuable tool for anyone seeking to make data-driven decisions.
Book a demo today to explore how Appinio can enhance your data collection and visualization efforts, ultimately empowering your decision-making process!